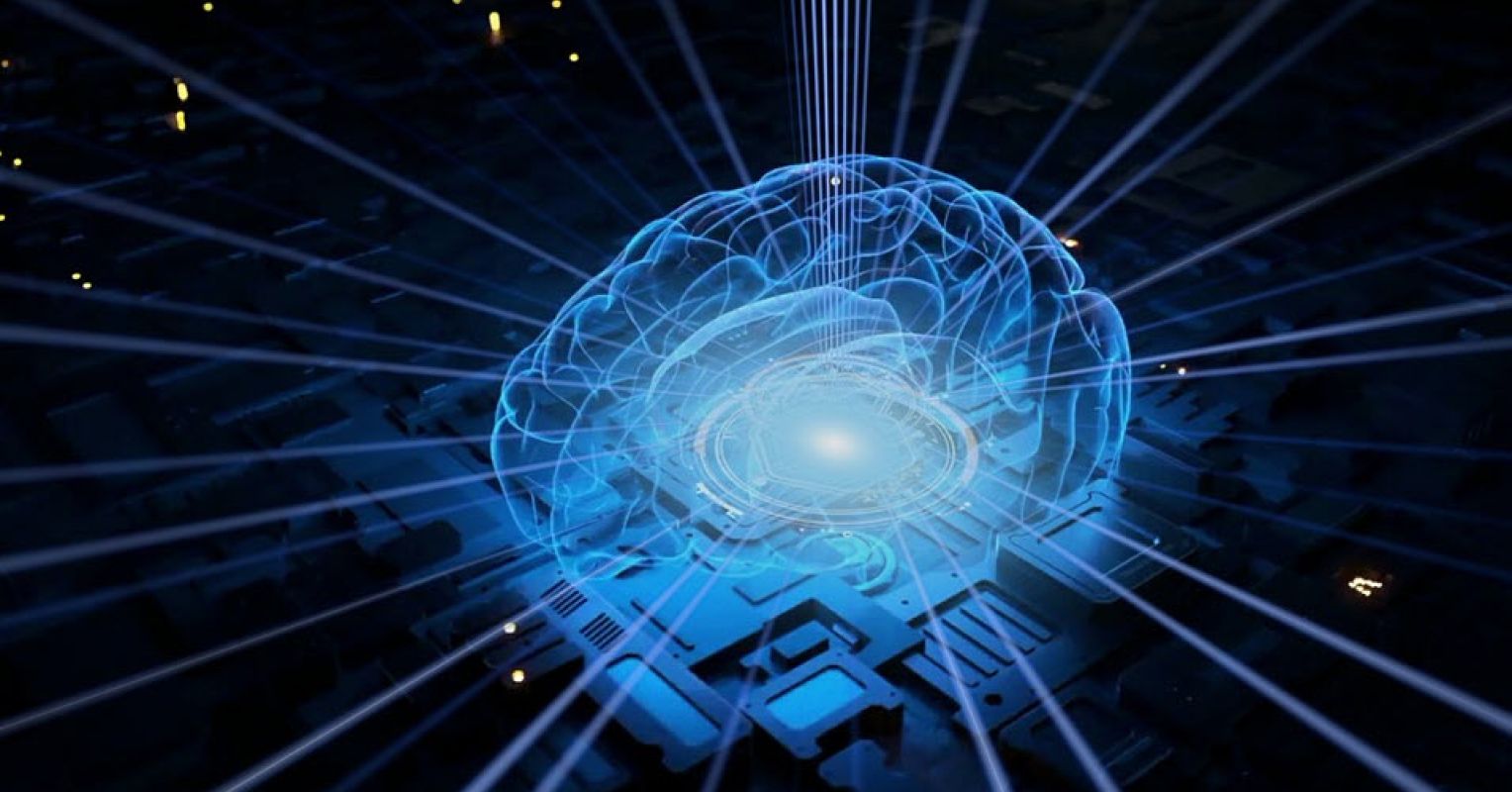
Mind-Influenced AI Liquid Neural Networks Electricity Drones
[ad_1]
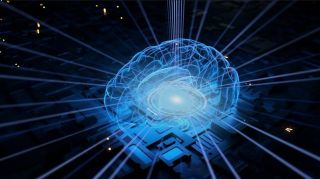
Placidplace/Pixabay
Artificial intelligence (AI) is enabling drones to carry out real-time analysis, mapping, and tracking of info from their cameras. Researchers from the Massachusetts Institute of Technologies (MIT) Laptop or computer Science and Synthetic Intelligence Laboratory (CSAIL) display how brain-encouraged AI liquid neural networks enable drones to navigate unfamiliar destinations in their analyze published this week in Science Robotics.
“The outcomes in this paper open the door to the risk of certifying equipment finding out answers for security important programs,” claimed senior co-creator Daniela Rus, Director at MIT CSAIL, and the Andrew (1956) and Erna Viterbi Professor of Electrical Engineering and Laptop Science at MIT.
Ramin Hasani is the analyze senior co-author and co-initially writer together with co-initially creator Makram Chahine, and researchers Alexander Amini, Mathias Lechner, Ryan Shubert, Aaron Ray, and Patrick Kao.
“Studying all-natural brains efficiently narrows the lookup space of attainable algorithms for obtaining clever actions,” wrote the researchers.
For this study, the MIT CSAIL researchers used liquid neural networks to make a learning-dependent solution to allow drones to navigate in unfamiliar environments. The inspiration for liquid neural networks is from organic organisms, namely, the microscopic nematode C. elgans, which can deliver intricate dynamics with just a minor around 300 neurons. This new course of AI algorithms was initial released in 2020 by Hasani and Lechner, alongside with Rus, Amini, and Radu Grosu.
Liquid time-continuous networks (LTCs) are a course of time-continual recurrent neural networks (RNNs). Contrary to standard synthetic neural networks, liquid neural networks take care of complexity improved and are more fluid, robust, expressive, and interpretable. In effect, liquid neural networks show artificial neuroplasticity. In typical neural networks, a fat reflects the toughness of the relationship among synthetic neurons. In distinction, in liquid networks, just about every neuron is managed by a nonlinear function which makes it possible for for variability hence it is a probabilistic approach.
The scientists executed a sequence of exams to see how properly the pre-experienced neural model could generalize when faced with facts it had not been qualified on prior. These shut-loop tests integrated flying to the focus on, flight vary, strain take a look at, attention profile of networks, goal rotation and occlusion, climbing with adversaries, a triangular multistep loop involving objects, and dynamic concentrate on monitoring.
The scientists in comparison 6 recurrent neural community architectures, which included temporal convolutional network (TCN), ODE–recurrent neural network (RNN), gated recurrent device (GRU)–ordinary differential equation (ODE), shut-sort ongoing-time (CfC), neural circuit coverage (NCP), and very long small-time period memory (LSTM). The shut-sort continuous-time and neural circuit policy algorithms were formulated by the MIT CSAIL scientists.
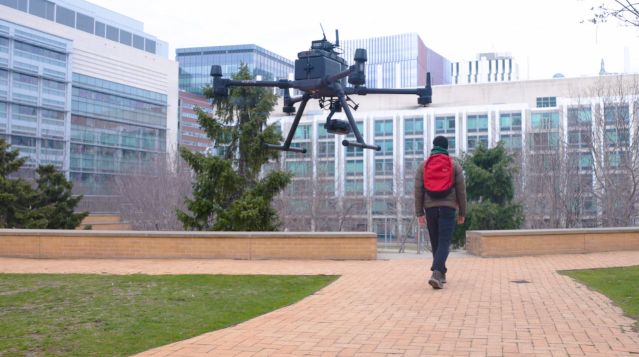
Resource: MIT CSAIL
Each and every algorithm product was examined in four environments consisting of city lawn, city patio, education woods, and alternative woods. In accordance to the scientists, their liquid neural networks were being able to generalize to new duties and novel environments much better than the well-liked condition-of-the-artwork recurrent neural networks.
“We had our good reasons to hope liquid neural networks would outperform other architectures on the fly-to-goal undertaking, based on the favorable homes inherent to them by structure,” explained Chahine. “But all that is on paper. I was really astonished to see how continuously they done flight navigation in the true environment. As the responsibilities we considered obtained much more and additional elaborate, only liquid networks held their floor, while all other condition-of-the-art architectures failed.”
Copyright © 2023 Cami Rosso All rights reserved.
[ad_2]
Resource link